In the fast-paced world of technology, the field of machine learning (ML) is experiencing continuous growth and evolution. Staying ahead of the curve by understanding emerging machine learning trends is crucial to unlocking its full potential.
This article explores the upcoming gadget mastering traits, along with accessibility, explainability, domain-unique packages, generative AI, and security and model. It offers insights into how device mastering is poised to revolutionize various components of our lives, providing treasured insights.
Democratization of Machine Learning: Empowering Everyone with Machine Learning Trends
The democratization of machine getting to know (ML) indicates a massive shift, making this effective technology more reachable and user-friendly than ever earlier. This fashion empowers people and agencies of all sizes to leverage the capability of ML without requiring significant technical knowledge, fostering innovation and trouble-fixing across numerous fields.
The rise of low-code/no-code systems is using the device-studying fashion, permitting users to build and set up models with intuitive interfaces, doing away with the want for complex coding, and empowering people with restrained technical backgrounds to participate in the ML revolution.
The democratization of machine learning has eliminated technology barriers, allowing a wider range of individuals to harness its potential. This encourages creativity, expands the range of possible uses, and sets the stage for a future in which becoming an expert gadget user won’t be exclusive to the realm of tech giants but rather will be a tool available to everyone.
Trust and Transparency: The Rise of Explainable AI (XAI) and Responsible AI
Explainable AI enhances confidence and transparency in machine learning by addressing concerns about the “black box” nature of the field.
XAI is a technology that aids in understanding and enabling humans to assess and potentially mitigate these biases. Interpretability and equity are crucial in device learning improvement, as biased records or algorithms can lead to discriminatory outcomes.
Responsible AI extends beyond XAI, focusing on moral concerns throughout the entire machine studying lifecycle. This encompasses accountable records collection practices, making sure information is privateness, and keeping off discriminatory biases in statistics choice. It also involves careful attention to capacity societal and moral implications throughout model education, deployment, and impact assessment. Adhering to hooked-up ethical frameworks and regulations performs an important role in making sure the responsible improvement and deployment of machine-mastering answers that gain society without unintentional results.
By embracing XAI and Responsible AI standards, we can foster trust and transparency in the device getting to know, ensuring its development and application align with moral values and foster positive societal effects.
Domain-Specific Machine Learning: Tailoring for Impact within the Machine Learning Trend Landscape
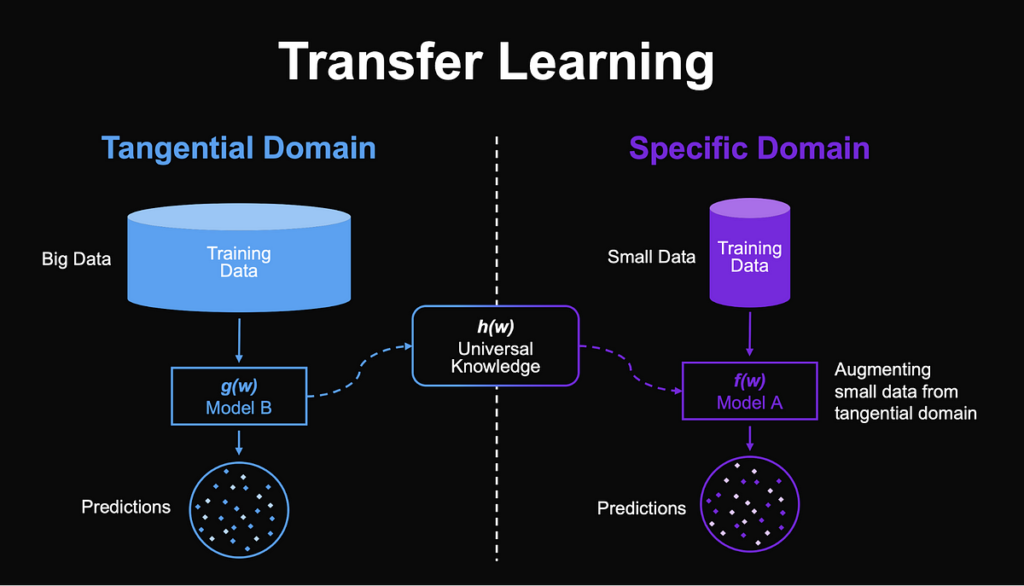
Domain-particular gadget gaining knowledge of tailors models to unique industries or domains, like healthcare or finance. This targeted technique in the broader machine-mastering fashion ends in numerous key advantages:
Improved performance: Models apprehend the hassle higher because of area-specific information and facts, leading to greater accurate and effective solutions.
Enhanced performance: Fewer data is wanted for schooling, making improvement and deployment more fee-effective, optimizing aid allocation inside the system getting to know the procedure.
Real-global applicability: Models tackle specific domain-specific challenges, ensuring their relevance and impact in the centered field.
The trend indicates a shift towards more impactful machine learning programs, promoting innovation and development across various sectors.
The Rise of Generative AI: Pushing Boundaries
Generative AI is a rising star in the machine learning trend landscape, generating new data and creative content like text, audio, or images. It unlocks possibilities in:
Content creation: Assisting creative fields with realistic content generation, inspiring artists, and personalizing experiences.
Drug discovery: Simulating molecules to accelerate drug development processes, potentially leading to faster medical breakthroughs.
Data augmentation: Artificially generating additional data to address limitations and improve the effectiveness of machine learning models.
While Generative AI holds immense potential, responsible development, and application are crucial to mitigate ethical concerns like misinformation or misuse. By prioritizing responsible practices, we can harness its potential for positive societal impact and creative exploration.
Security in Machine Learning: A Growing Priority
As device learning adoption surges, so does the point of interest in its safety. Key worries consist of:
Data protection: Protecting touchy statistics used to educate and install fashions, safeguarding privacy, and stopping unauthorized access.
Model safety: Ensuring the integrity and robustness of fashions towards manipulation or assaults, preserving the reliability of their predictions.
Implementing robust safety features is crucial to deal with those worries and protect the integrity and reliability of device studying systems, fostering stable and honest programs across various fields. Democratization, explainability, generative AI, security, and continuous learning: discover the hottest machine learning trends shaping the future!