In the digital age, cyber threats which include ransomware, and professional phishing present extreme risks to people and corporations. Cybersecurity professionals are finding that device-gaining knowledge is a useful tool for keeping up with those improvements. This article explores the transformative capabilities of machine learning in cybersecurity and predicts future threats.
Understanding Machine Learning in Cybersecurity:
Large volumes of statistics are utilized to learn and provide a baseline for common pastimes. Security experts can have a look at in addition using flagging anomalies, or departures from this baseline, as possible risks. Compared to conventional signature-based total detection, which depends on pre-described styles of diagnosed threats, this approach has numerous blessings. Because gadget studying is flexible and can recognize new assault strategies, it’s far a critical device within the constantly changing global cyber threats.
Benefits of Machine Learning in Cybersecurity:
Machine studying offers a large number of advantages that are revolutionizing the way corporations method cybersecurity.
Enhanced Threat Detection: Unlike traditional methods, machine learning doesn’t rely on pre-defined signatures. Instead, it analyzes complex patterns and relationships within data, enabling it to identify even the most sophisticated, zero-day attacks with impressive accuracy, significantly reducing the risk of successful breaches.
Improved Efficiency: By automating tedious duties like log file evaluation and malware detection, system studying frees up precious time and assets for safety employees. This lets them to cognizant of more strategic tasks, including vulnerability assessment and threat looking, leading to an improved security posture.
Continuous Learning and Adaptation: Unlike static signature-based total structures, devices gaining knowledge of models constantly examine and adapt to the ever-evolving cyber risk panorama. This guarantees they remain effective towards new attack vectors and rising threats, imparting a future-proof answer for robust cybersecurity.
Applications of Machine Learning in Cybersecurity:
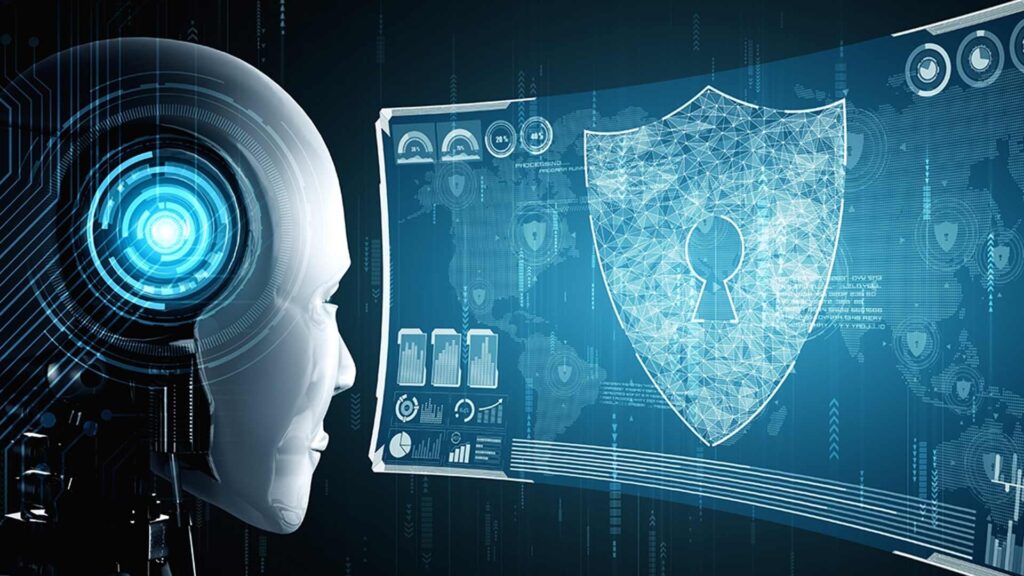
Machine getting to know performs a crucial role in diverse cybersecurity programs, empowering businesses to guard against a diverse variety of threats:
Malware Detection: The machine getting to know can identify malicious software programs like viruses, worms, ransomware, and spyware by way of reading document conduct, network pastime, and code patterns. It uses techniques like static and dynamic analysis to look at code shape and hit upon state-of-the-art malware in real-time.
Intrusion Detection and Prevention (IDS/IPS): IDS/IPS structures can now analyze network visitors for abnormalities and suspicious interest ways to get to know. If an incursion is suspected, those systems might also stumble on it, notify the safety body of workers, and in the case of intrusion prevention structures, even actively save you harmful efforts. By being proactive, the danger of unauthorized access to vital structures and information is substantially decreased.
Phishing and Social Engineering Attack Detection: As social engineering approaches become more sophisticated, device mastering remains an important defense. It analyzes email content, sender statistics, and language patterns to pick out suspicious characteristics. For example, gadget learning can come across subtle inconsistencies in electronic mail language, unusual sender addresses, or tries to impersonate legitimate senders, assisting individuals and corporations to keep away from falling victim to phishing scams.
User and Entity Behavior Analytics (UEBA): By employing system mastery, UEBA is beyond basic customer activity monitoring. It looks at the relationships and context between various machine elements, such as users, devices, and software. UEBA may also identify potential attacks, such as insider threats and compromised invoices, by identifying deviations from normal behavior patterns. Protection teams can use UEBA to identify unusual access attempts from unexpected places, attempts to steal data, or sudden changes in individual activity patterns to detect and prevent security breaches.
Machine learning is revolutionizing cybersecurity in diverse approaches, with future trends predicting greater advanced applications to beautify defenses towards the growing chance of cyberattacks.
Challenges:
Data Quality: Biased data can lead to unfair outcomes. Careful data selection and monitoring are crucial.
Explainability: Complex models can be difficult to understand. Explainable AI techniques can help build trust.
Ethics: Data privacy is a major concern. Robust data governance practices are essential.
While machine learning offers vast potential, addressing these challenges is crucial for responsible and ethical implementation in cybersecurity.
The Future of Machine Learning in Cybersecurity:
Machine-gaining knowledge of advancements, consisting of unsupervised and deep getting-to-know, can revolutionize cybersecurity. Integrations with blockchain and cloud protection provide greater robust protection mechanisms. However, continuous mastering and modeling from both fashions and safety experts are crucial to staying vigilant towards evolving cyber threats.
Conclusion
In the digital age, machine learning is an essential tool for thwarting cyberattacks. Companies should adopt it sensibly, taking into account issues like explainability, data quality, and moral issues. Maintaining an edge over the constantly changing environment of cyber threats requires constant learning and change. Examine today’s ways that machine learning might strengthen cybersecurity defenses for a safe tomorrow.